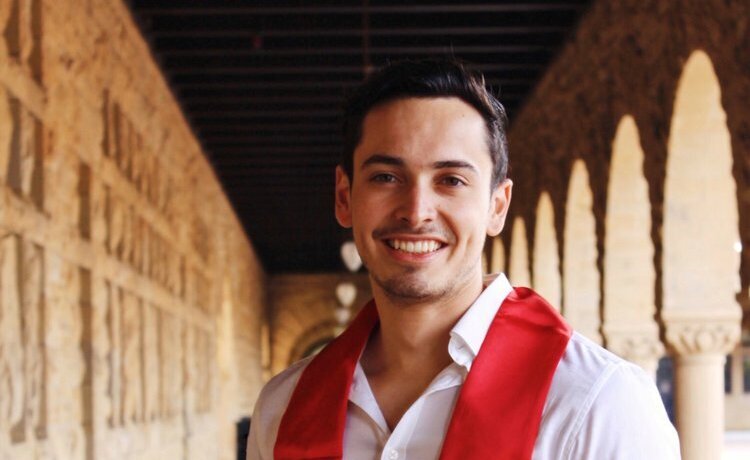
Richard Diehl Martinez is interested in teaching Artificial Intelligence to learn in a more human way so it can adapt to new tasks
I am interested in how we take inspiration from how humans learn and code it into an AI model.
Richard Diehl Martinez
The last year has seen an explosion in generative AI, particularly ChatGPT, with warnings about job losses and cheating in exams, but also excitement about the possibilities it offers to do things faster and more efficiently.
However, despite concerns about bias and other ethical issues, there has not been as much discussion of the model it is built on and what that could mean for the information we rely on in the future.
Simply put, generative AI draws on big data, the kind of big data that is usually concentrated in the hands of major companies. For Richard Diehl Martinez [2021], who has worked in AI both from an academic as well as an industry perspective, this presents a lot of concerns, for instance, when it comes to languages – a subject close to his heart given he grew up speaking three and being exposed to endangered languages in Guatemala.
English absolutely dominates the big data pile and minority or endangered languages where there is little written data almost don’t feature.
That bias is also true for research – research published in the English language will dominate a big data approach. Yet humans don’t learn in this quantitative way. They are more flexible and can adapt their learning to other contexts.
Richard says: “I am interested in how we take inspiration from how humans learn and code it into an AI model.” He adds: “The aim is not to train AI to do well in one given task, but to train it instead to be a good learner so it can adapt its learning model to new tasks.”
It’s an ambitious task which draws not only on his own upbringing and interests, but on his multidisciplinary background.
Early life
Richard was born in Boston, but grew up between the US, Guatemala and Germany. His father is from Germany and mother is from Guatemala. The family moved to her home town in a rural part of the country when Richard was little. His first years of school were spent there, surrounded by his large extended family. It ignited his interest in language. There were many indigenous people in the community.
In fact, Richard discovered he had Mayan descendants. His grandfather would often tell him that when he was a child he used to speak lots of different Mayan languages that had since disappeared. He taught Richard some Mayan words. “It was the start of my interest in discovering different languages and my culture,” he says.
When Richard was nine the family moved back to Boston and then left again for Germany just over two years later. Richard attended the John F Kennedy School in Berlin where students could take either US or German qualifications. Half the teachers were German and half from the US. As Richard initially wanted to go to a European university, he started down the German qualification track, but then changed his mind because he felt the US system offered more time for him to decide where he wanted to focus.
Living and going to school in three different countries gave him an insight into how different education systems work. The German system was strong on social sciences and humanities and Richard left school thinking he would study politics and economics at university. At school he was very involved with the mock UN, becoming Secretary General, and attended a lot of conferences.
Stanford
He applied to study political science at Princeton, but on a whim he also applied to Stanford and was blown away by a visit there. “I thought maybe I am interested in science,” he says. He started at Stanford, intending to pursue a degree in international relations. However, in his second year he was working as a research assistant in a laboratory where he was constructing financial models for East Asia, looking at the relative costs and long-term outcomes of, for instance, health and education initiatives. To do this he had to learn how to programme and how to analyse statistics so he took some computer science and statistics courses and found he really enjoyed them and the collaborative nature of computer science.
By his third year he had to pick a major and opted to do one in management sciences and engineering, a unique major at Stanford. Many on the course went on to work in consulting, but that was not a path that interested Richard. He loved the ability to create simulations and to explore on his own. On finishing his degree in 2018, he decided therefore to stay on to do a master’s to expand his knowledge of computer science and learn more about how computers work in order to integrate his interest in languages, politics and social science with computer science.
Richard was aided in his explorations by his mentor Professor Dan Jurafsky, one of the leading thinkers in how Artificial Intelligence is applied to languages. Professor Jurafsky was interested in looking at bias and specifically in how to create AI that can counter bias. It was the time of the 2016 presidential election in the US and there was a lot of discussion about how to define political bias. “It forced you to think very deeply about the purpose of AI models. It was very philosophical,” says Richard. “I loved it. During that process I realised that my interest was in natural language processing – how AI is applied to language.”
Richard worked on two projects with Professor Jurafsky which looked at AI from a social and computational basis and published several academic papers, including one on detecting bias. He finished his master’s in 2020 and decided that, although he wanted to do a PhD, it would be good to spend some time in industry working on a big project to flex both his engineering and computer science skills.
Alexa
He sought out companies that were interested in research on natural language processing. Most companies required their researchers to have a PhD, but the Alexa team at Amazon allowed Richard to do research that was allied to his master’s work. He moved to Seattle and stayed with Amazon for a year and a half, working in a big team, mainly from home during the pandemic. “It felt like I was back at university but being paid, working on problems on a larger scale that could impact millions of people,” he says.
Richard had to pitch his research ideas to his team manager and the best ideas were developed further. One of his was given the green light and he started working on it with an engineering team. It involved greater personalisation of Alexa so the AI could respond based on particular knowledge of the customer, such as their work patterns or when they woke up. For instance, if the person used Alexa mainly in the mornings it could be inferred that it was used as an alarm and be primed for that. The changes involved using linear algebra methods based on the person’s previous interactions with Alexa.
By the end of 2021, Richard was keen to pivot away from natural language processing problems. AI was progressing rapidly with the foundations of ChatGPT being built based on big data and computational power. “I was feeling frustrated that the field was all about drawing on more data without thinking about how to improve the models from a mathematical sense,” he says.
Cambridge
He felt his only option was to find the kind of niche research laboratory that focused more on this or do a PhD. He asked Professor Jurafsky for advice and he suggested speaking to Professor Paula Buttery, who was a visiting professor at Stanford at the time and is now his supervisor. Her research looks at modelling AI to how children learn language. It seemed a natural fit for Richard.
“My research looks at how we can make the language models AI draws on more human like so they can learn with less data and computational intensity,” he states. “I want to think about the more fundamental properties of how to train AI in a more human-like way.” His end goal is to be able to teach AI to learn languages where there is not a lot of textual or spoken data.
His PhD has been focused on three different perspectives – the model, the data that feeds it and the training paradigm through which the model learns and gets smarter.
For the first two years he focused on the learning paradigm and how to move from the big data model to a smaller, smarter model based on meta learning. He hopes this will address some of the bias in AI. Richard, who is in his third year, is looking forward to presenting his model in Singapore and is hoping to continue in fundamental research about creating language models with less bias.